Some practical tips to use AI with your team
The use of large language models (“LLMs”) such as ChatGPT can be a controversial topic. People have strong opinions about them for all kinds of reasons, from concerns around data privacy to the propagation of bias and inequality. The amount of energy used to train LLMs, and the amount of water used to cool data centres on which cloud-based versions run, is a particular concern. Our project with Friends of the Earth around Harnessing AI for environmental justice starts to unpack some of the environmental complexities of AI.
However, LLMs can be really useful as well, so in this post, we’re going to take a nuanced look at how various types of LLMs can be used together to improve both process and outcomes. We believe this is in keeping with the Spirit of WAO and our five focus areas, we believe in dialogue that leads to action. At WAO we’ve had a number of conversations around our personal use of AI and how we prefer to use it in our collective work.
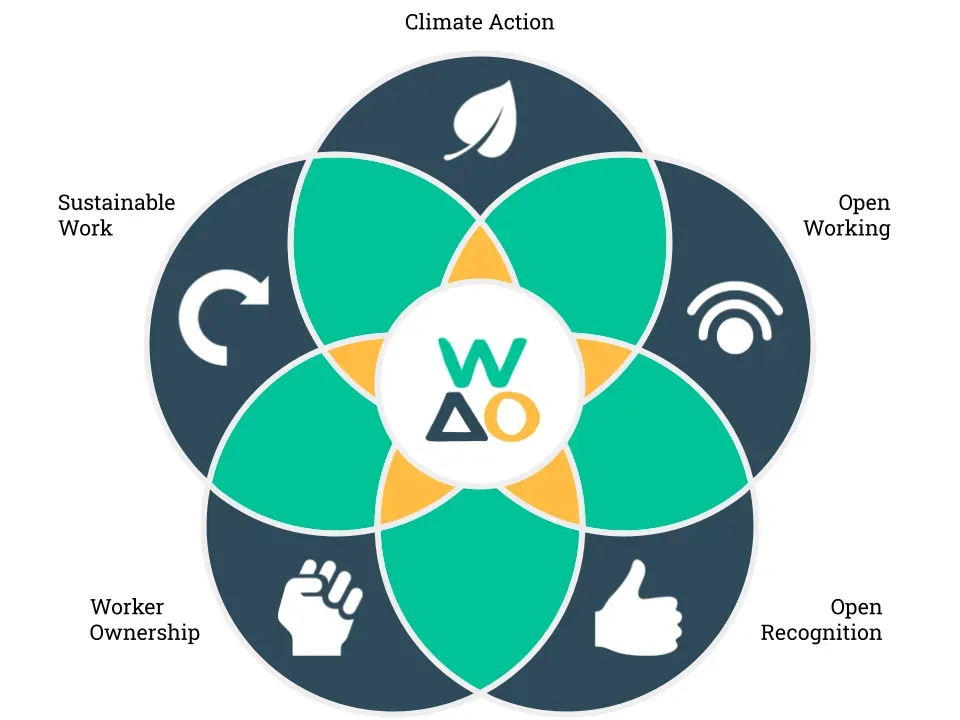
Why use LLMs?
Having used them regularly as part of our daily work for over 18 months at this point, we can say that using LLMs is useful for us in a number of concrete ways:
- Speed — outsourcing ‘grunt’ work to LLMs means that we can spend time doing more creative work. A good example of this is using the optical character recognition (OCR) capabilities of some LLMs to recognise virtual sticky notes from project pre-mortem sessions we run with clients. It can then turn these into text, categorise them, and produce a spreadsheet. What would take perhaps an hour can be done in a couple of minutes.
- Situational perspectives — Asking LLMs to perform a ‘red/amber/green’ or RAG analysis of work can also be useful. It’s easy to get carried away with personal interests or to miss an important part of a client brief. By asking LLMs to check your work using the RAG format against what has been requested, we can check that our work fits with what’s required. It can also help us think through problems: on Doug’s personal blog he discussed how he used an LLM in the process of deciding not to go through with a house purchase. The AI helped calculate things such as cumulative risk of flooding over time, using formulae he might not have otherwise known how to use.
- Text synthesis — LLMs are excellent at synthesising information quickly and efficiently. This is true of the recently-launched Google NotebookLM which allows users to share up to 50 data sources, synthesise and then query them. One important point, however, is that we need to be thoughtful and focused in checking the accuracy of synthesis and summarisation work because LLM’s are also great at hallucinating.
Three ways to approach LLMs sustainably
Start local
There’s a lot to unpack around the use of AI and the environmental impacts. As a tech and environmental activist, I was hesitant about using some of the popular, browser-based LLMs for a variety of reasons. I recently wrote up a quick, Captain Planet themed overview of some of the climate crisis issues that this kind of technology contributes to. Together with WAO members, I’ve discussed and debated various privacy, bias and attribution issues that are baked into our technology choices.
Having started to use AI across a lot of contexts and looking into the impacts that the use of AI has, we made the decision to use locally installed models and tools to help reduce our climate impact whenever possible. Locally installed models might not be updated as frequently as ChatGPT, but the climate benefits are huge. The processing happens locally, as does the storage, so your queries and conversations aren’t stored in big data centres and they remain private to you.
Use multiple models
There are many generative AI models, some of which are smaller and some which are larger. A good place to see the diversity of these models is Hugging Face, a slightly awkwardly-named AI community. Here you can find the latest versions of models, along with datasets, and implementations of AI for various purposes.
It can be complicated to use the command line to install, configure, and run LLMs locally. However, as macOS users one of the tools we’ve been playing with is RecurseChat which simplifies the use of multiple, local AI models within an easy-to-use app. It’s straightforward to install and has a privacy policy that doesn’t keep us up at night worrying about where our data is going.
Conduct experiments
When AI image generation first hit the scene, we were excited about its possibilities. Together, we made art projects like Time’s Solitary Dance or the PsychOps for Mental Health Awareness month, which I made pairing AI generated images with the fabulous Remixer Machine.
While we certainly had fun playing with image generation, we now default to simply searching for images (or asking Bryan Mathers to draw them for us ;) There is an incredible emission cost to image generation, so we think carefully before asking generative AI to make something for us.
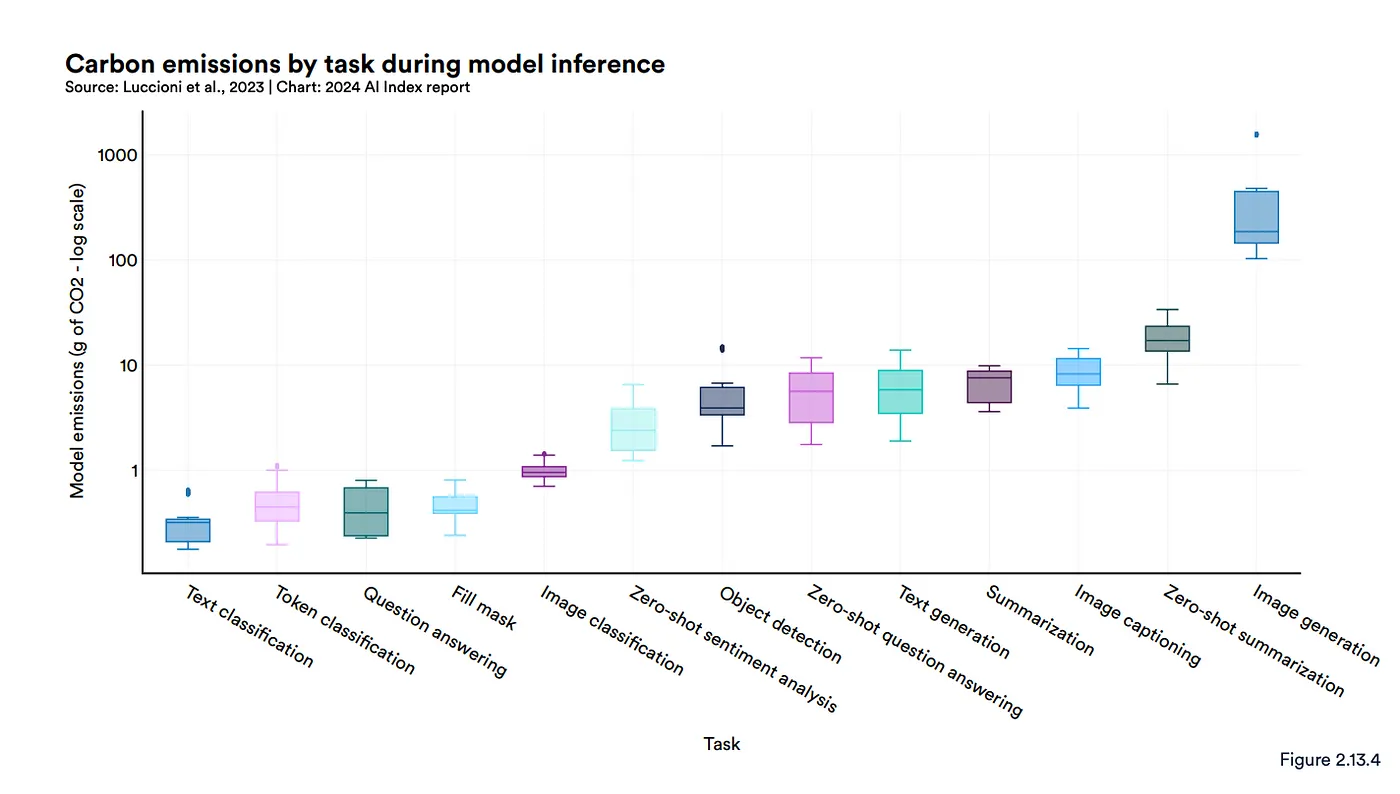
Being environmentally conscious involves uncovering a great many complexities within our world. For example, when talking about the overall consumption of energy and water people tend to be quite alarmist. After a conversation with one of our gaming buddies about the annual energy use of a beer fridge, we calculated that it was the equivalent of prompting ChatGPT 4 over 2,200 times. Similarly, we calculated that you could prompt over 300 times for the same water usage as washing a car with a hose. While we recognise that using AI is an additional resource use, we try to put it in the context of wider consumption patterns.
Go forth and prompt together
If you are working alone and making use of LLMs, be sure and let people know what your “little robot friend” had to say. At WAO we let each other know when something we’re pasting into a chat is AI generated or if we’re about to prompt an LLM on something. Transparency is an important part of the process.
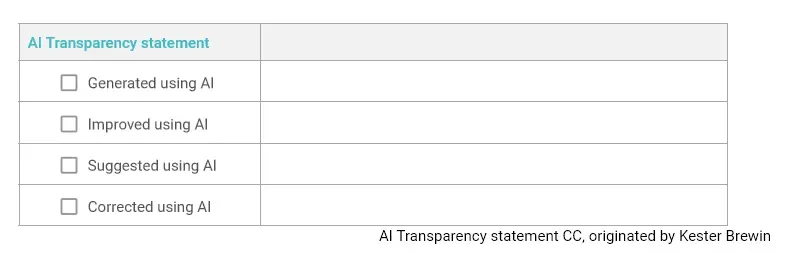
Part of AI literacy is being able to spot common foibles of LLMs. For example, we’ve come to dislike the words ‘delve’ and ‘foster’ because of their overuse by ChatGPT. Once you start noticing particular models tend towards certain words or phrases, you start seeing them everywhere. This is another reason transparency is so important.
Using a local model and screen sharing while coworking with your team is a great way to learn about AI together. You can use AI prompting and team conversation to bounce ideas around and find some productive ambiguity. Another benefit of this process is, of course, the laughter. AI responds with some pretty hilarious things sometimes, so why not improve your team dynamic by making fun of AI together.
Do you need help with this kind of thing? Get in touch with WAO!